Supporting Evidence-based Public Health Interventions using Text Mining
Introduction
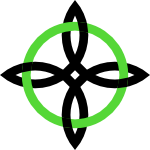
This project aims to conduct novel research in text mining and machine learning to transform the way in which evidence-based public health (EBPH) reviews are conducted. The project is a collaboration between three institutions:
- The National Centre for Text Mining (NaCTeM), with its proven track record of developing effective text mining tools operating in a variety of domains.
- The Machine Learning and Data Analytics (MaLDA) at the University of Liverpool, specialising in the application of machine learning, data mining and general mathematical modelling and optimisation methodologies to complex real-world problems.
- The National Institute for Health and Care Excellence (NICE), the world's leading centre of the development and application of the principles of evidence-based medicine to technology appraisal, clinical guidelines and public health.
Project goals
- to develop new text mining unsupervised methods for deriving term similarities, based on distributional semantics, to produce meaningful and high quality document and label clusters to support screen while searching in EBPH reviews.
- to develop new seriation algorithms for ranking and visualising meaningful associations of multiple types, dynamically and iteratively.
- to evaluate these newly developed methods in EBPH reviews, based on implementation of a pilot, to ascertain the level of transformation in EBPH reviewing.
RobotAnalyst
RobotAnalyst is a new tool that builds upon state of the art text mining technologies, including topic modelling and feedback-based text classification models, to minimise the human workload involved in the study identification phase.News
13th July 2017
NaCTeM is organising two workshops at the Global Evidence Summit, to be held in Cape Town, South Africa from 13th - 16th Sept 2017. The workshops will be entitled RobotAnalyst: an online system to support citation screening in evidence reviewing and Screening evidence for systematic reviews using a text mining system: the RobotAnalyst
12th July 2017
John McNaught gave a talk at the Text and Data Mining Symposium, held at the University of Cambridge on Wednesday 12th July 2017.
22nd June 2017
Prof. Sophia Ananiadou gave an invited talk at the University of Cambridge on 23rd June 2017, as part of the PulblicHealth@Cambridge series of seminars. The talk was entitled Text mining for public health reviews (The Robot Analyst).
5th October 2016
Prof. Ananiadou discussed the work carried out on this project during her participation in a panel session entitled Evidence Synthesis - Current Practices and Future Possibilities to be held as part of the IEEE International Conference on Healthcare Informatics (ICHI 2016), in Chicago, IL, USA.
6th June 2016
NaCTeM organised a workshop at the 24th Cochrane Colloquium, Seoul, Korea, to be held from 23rd -27th October 2016. The workshop was entitled Text mining methods to support the development of sensitive search strategies in public health reviews, and was organised in collaboration with the Public Health and Social Care Centre at the National Institute for Health and Care Excellence (NICE).
20th May 2016
The project is mentioned in a new article about text mining and the work of NaCTeM, published in Pharma Technology Focus, a bi-monthly magazine that brings together the latest insights and innovations from across the pharaceutical industry.
3rd October 2015
NaCTeM attended the 23rd Cochrane Colloquium, held in Vienna, Austria from the 3rd - 7th of October 2015 and co-organised a workshop session entitled The present and future use of text mining for study identification on the 5th of October.
Project information
The project is funded by the Medical Research Council for a period of 3 years, starting from 31st March 2014 (Grant No. MR/L01078X/1).Project team
Prinicpal Investigator: Prof. Sophia Ananiadou (NaCTeM)Co-Investigators: Mr. John McNaught (NaCTeM), Dr. John Goulermas (MaLDA).
Researchers: Dr. Austin Brockmeier (NaCTeM), Dr. Piotr Przybyla
Related Publications
Mu, T., Goulermas, J. Y and Ananiadou, S. (2017). Data Visualization with Structural Control of Global Cohort and Local Data Neighborhoods IEEE Transactions on Pattern Analysis and Machine Intelligence, 99
Kontonatsios, G., Brockmeier, A. J., Przybyla, P., McNaught, J., Mu, T., Goulermas, J. Y and Ananiadou, S. (2017). A semi-supervised approach using label propagation to support citation screening. Journal of Biomedical Informatics 72, 67-76.
Sato, M., Brockmeier, A. J., Kontonatsios, G., Mu, T., Goulermas, J. Y, Tsujii, J. and Ananiadou, S. (2017). Distributed Document and Phrase Co-embeddings for Descriptive Clustering. In Proceedings of EACL, pp. 991 - 1001.
Alnazzawi, N., Thompson, P. and Ananiadou, S. (2016). Mapping Phenotypic Information in Heterogeneous Textual Sources to a Domain-Specific Terminological Resource. PLOS ONE, 11(9), e0162287
Haynes, C., Kay, N., Harrison, K., McLeod, C., Shaw, B., Leng, G., Kontonatsios, G. and Ananiadou, S. (2016). Using text mining to facilitate study identification in public health systematic reviews. In: Guidelines International Network (G-I-N) conference
Hashimoto, K., Kontonatsios, G., Miwa, M. and Ananiadou, S. (2016). Topic Detection Using Paragraph Vectors to Support Active Learning in Systematic Reviews. In: Journal of Biomedical Informatics, 62, 5965
Mo, Y., Kontonatsios, G. and Ananiadou, S.. (2015). Supporting Systematic Reviews Using LDA-based Document Representations. Systematic Reviews , 4, 172
Alnazzawi, N., Thompson, P., Batista-Navarro, R. and Ananiadou, S. (2015). Using text mining techniques to extract phenotypic information from the PhenoCHF corpus. BMC Medical Informatics and Decision Making 15 (Suppl. 2):S3 .
Miwa, M. and Ananiadou, S. (2015). Adaptable, high recall, event extraction system with minimal configuration. BMC Bioinformatics 16:(Suppl. 10):S7
Xu, Y., Chen, L., Wei, J., Ananiadou, S., Fan, Y., Qian, Y., Chang, E. I-C. and Tsujii, J. (2015). Bilingual term alignment from comparable corpora in English discharge summary and Chinese discharge summary. BMC Bioinformatics 16:149
O'Mara-Eves, A., Thomas, J., McNaught, J., Miwa, M. and Ananiadou, S. (2015). Using text mining for study identification in systematic reviews: A systematic review of current approaches. Systematic Reviews 4:5 (Highly Accessed)
Mu, T., Goulermas, J. Y, Korkontzelos, I. and Ananiadou, S. (2014). Descriptive Clustering via Discriminant Learning in a Coembedded Space of Multi-level Similarities. Journal of the Association for Information Science and Technology
Ananiadou, S., Thompson, P., Nawaz, R., McNaught, J. and Kell, D. B. (2014). Event Based Text Mining for Biology and Functional Genomics. Briefings in Functional Genomics
Miwa, M., Thomas, J., O'Mara-Eves, A. and Ananiadou, S. (2014). Reducing systematic review workload through certainty-based screening. Journal of Biomedical Informatics
Miwa, M., Thompson, P., Korkontzelos, I. and Ananiadou, S. (2014). Comparable Study of Event Extraction in Newswire and Biomedical Domains. In Proceedings of Coling 2014
Xu, Y., Hua, J., Ni, Z., Chen, Q., Fan, Y., Ananiadou, S., Chang, E. I-C. and Tsujii, J. (2014). Anatomical entity recognition with a hierarchical framework augmented by external resources PLOS ONE, 9(10), e108396
Further information
- Connect to us on Twitter.
- Contact us: Prof. Sophia Ananiadou.
Featured News
- AI for Research: How Can AI Disrupt the Research Process?
- ELLIS Workshop on Misinformation Detection - Presentation slides now available
- 1st Workshop on Misinformation Detection in the Era of LLMs (MisD)- 23rd June 2025
- Prof. Sophia Ananiadou accepted as an ELLIS fellow
- Invited talk at the 15th Marbach Castle Drug-Drug Interaction Workshop
- BioNLP 2025 and Shared Tasks accepted for co-location at ACL 2025
- Prof. Junichi Tsujii honoured as Person of Cultural Merit in Japan
- Participation in panel at Cyber Greece 2024 Conference, Athens
- New Named Entity Corpus for Occupational Substance Exposure Assessment
Other News & Events
- CL4Health @ NAACL 2025 - Extended submission deadline - 04/02/2025
- Shared Task on Financial Misinformation Detection at FinNLP-FNP-LLMFinLegal
- FinNLP-FNP-LLMFinLegal @ COLING-2025 - Call for papers
- Keynote talk at Manchester Law and Technology Conference
- Keynote talk at ACM Summer School on Data Science, Athens